The Role of Data Analysts in Informed Decision-Making
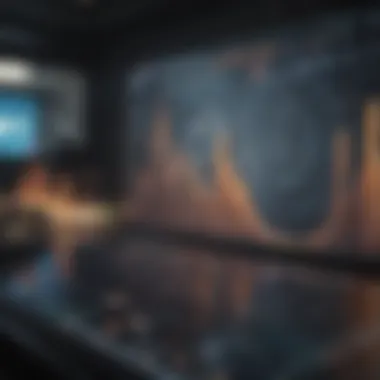
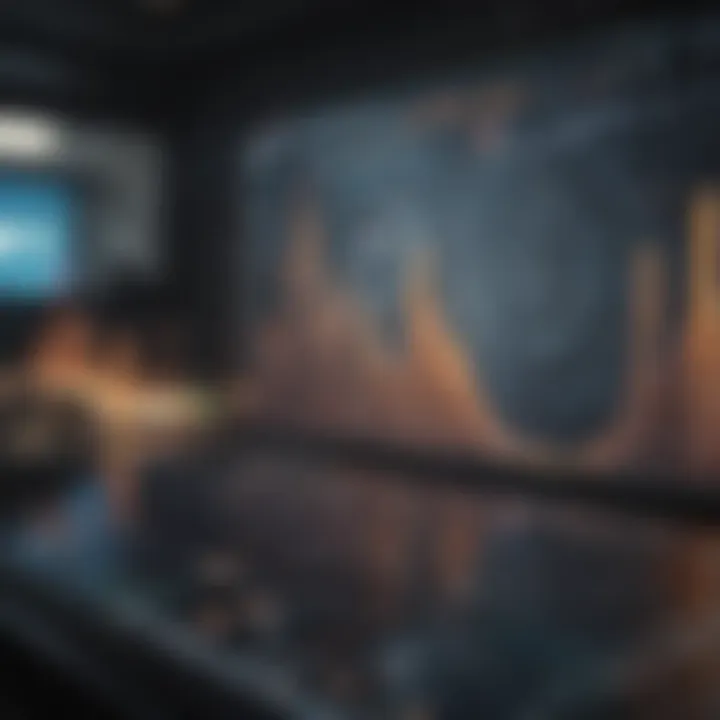
Intro
In today’s fast-paced business environment, making informed decisions is crucial. One role that stands at the forefront of this data-driven landscape is that of a data analyst. Organizations across various sectors rely on data analysts to sift through mountains of raw information, interpreting complex datasets to arrive at actionable insights. With the sheer volume of data generated daily, having skilled analysts can be the difference between navigating a successful strategy or hitting a brick wall.
Data analysts are not just number crunchers; they are storytellers who weave narratives from figures. Through their eyes, businesses can see trends, understand customer behaviors, and ultimately shape strategies that align with their goals. But what exactly do we mean when we talk about data analysis? Let’s break it down.
Understanding the Role of a Data Analyst
In today's fast-paced business environment, the role of a data analyst has become a linchpin in decision-making processes. Organizations are drowned in an avalanche of data, coming from various channels—be it social media, customer transactions, or market trends. This deluge of information necessitates skilled individuals who can sift through the noise to discover actionable insights. Understanding the role of a data analyst is vital for emphasizing how they bridge the gap between raw data and strategic decision-making.
Data analysts translate numbers and statistics into compelling narratives that any stakeholder can grasp. Their significance lies not just in handling data, but in their ability to interpret it in a meaningful way. They empower decision-makers by providing clarity and direction, which is crucial in an age where every move counts. This article addresses the intricacies of data analysis, highlighting how these professionals affect companies across various sectors by enhancing efficiencies and fostering informed decisions.
Defining Data Analysis
At its core, data analysis involves a systematic processing of data to extract useful information. The nature of data analysis can be categorized broadly into three types: descriptive, predictive, and prescriptive analysis. These processes enable businesses to identify trends, make forecasts, and even prescriptively recommend actions. Understanding this framework is beneficial as it lays the groundwork for how data can be utilized to support strategic objectives.
Core Responsibilities of Data Analysts
Data analysts wear many hats, and their responsibilities extend far beyond mere number-crunching. They are key players in the decision-making tapestry of modern organizations.
Data Collection and Preparation
Data collection and preparation serve as the bedrock of effective data analysis. This phase involves gathering data from diverse sources and formatting it for analysis. Analysts must ensure that the information they collect is relevant, comprehensive, and accurate. A significant characteristic of this stage is that it requires a keen eye for detail. This meticulousness is beneficial as it reduces potential errors in later analysis phases. However, the challenge lies in the compatibility of data from various sources, which may require extensive adjustments to harmonize.
Data Cleaning and Validation
Once the data is collected, the next step is data cleaning and validation. This process is crucial, as it addresses inconsistencies or inaccuracies that can skew results. A core attribute of data cleaning is its iterative nature—analysts check and re-check their datasets to eliminate any anomalies. This step is not just an annoying hurdle; it is vital for maintaining the integrity of the findings. While it demands a significant investment of time and resources, the advantages far outweigh the disadvantages, as clean data leads to more reliable insights and, ultimately, better decisions.
Data Visualization Techniques
Data visualization techniques play a key role in making complex datasets accessible to a broader audience. By employing charts, graphs, and dashboards, data analysts transform intricate data points into visual narratives that simplify understanding. This characteristic is essential as it aids stakeholders in grasping insights at a glance, promoting quicker decision-making. The unique advantage of data visualization lies in its ability to highlight trends and patterns that might be invisible in raw data. While effective visualizations can be compelling, analysts must also be cautious not to obfuscate the data's integrity in pursuit of aesthetic appeal.
"Good data visualization helps to build a bridge between the findings of sophisticated analysis and its practical implications for decision-making."
In essence, understanding the role of data analysts dives into their world of data collection, cleaning, and visualization. Each of these components interlinks, forming the foundation of effective decision-making within organizations. This broad view not only elucidates their importance but also provides insight into how their skill sets are integral to modern business operations.
The Importance of Data in Decision Making
In an age where information is more abundant than water in a river, understanding the role of data has become paramount in steering decision-making processes across various sectors. Organizations, big and small, harness the power of data not merely as a collection of numbers but as a compass guiding their strategic maneuvers. In this landscape, data analysts emerge as invaluable allies, unraveling the stories hidden within data sets and providing actionable insights that influence both daily operations and long-term goals.
Data as a Strategic Asset
Data is not just a byproduct of business activities; it's a strategic asset that can dictate the success or failure of an organization. This perspective has shifted the way firms view data—from being seen as a mere operational detail to being integral to fostering competitive advantage.
For instance, companies like Amazon have mastered the art of using data to optimize their operations. By analyzing purchase histories and customer preferences, they predict what products will fly off the shelves next. This capability allows them to tailor their stock, ensuring that they not only meet customer demand but also maximize profits. The insights drawn from data can significantly influence everything from marketing strategies to product development.
Moreover, the strategic use of data helps organizations mitigate risks. In the financial sector, for instance, data analysts employ predictive models that can forecast market trends and fund performances. By leveraging data, they provide evidence-based recommendations that support sound investment decisions, ultimately safeguarding investors' interests.
"In today's digital economy, data is like oil—it’s incredibly valuable when refined and utilized effectively."
The nuances in interpreting data cannot be understated. A company's ability to translate raw numbers into meaningful narratives is often what distinguishes successful enterprises from those that struggle. This process demands a unique blend of technical prowess and analytical thinking, underscoring the need for skilled data analysts.
How Data Drives Business Growth
At its core, data is about discovery. It illuminates paths that organizations may not have considered, providing clarity in a world full of uncertainty. The influence of data on business growth is multifaceted, impacting everything from operational efficiencies to customer engagement strategies.
One of the primary ways that data contributes to business expansion is through targeted marketing. Companies use data analytics to create deeply personalized marketing campaigns that resonate with individual consumers. For instance, Spotify utilizes listening data to recommend playlists, fostering user engagement and enhancing customer loyalty. When marketers harness data effectively, they can convert insights into actions that attract and retain customers more adeptly than ever before.
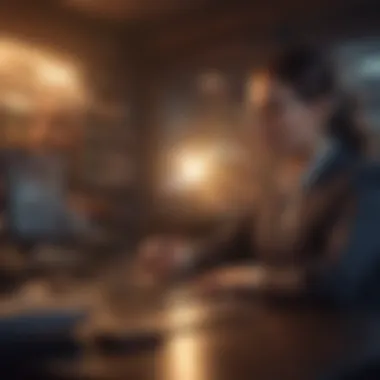
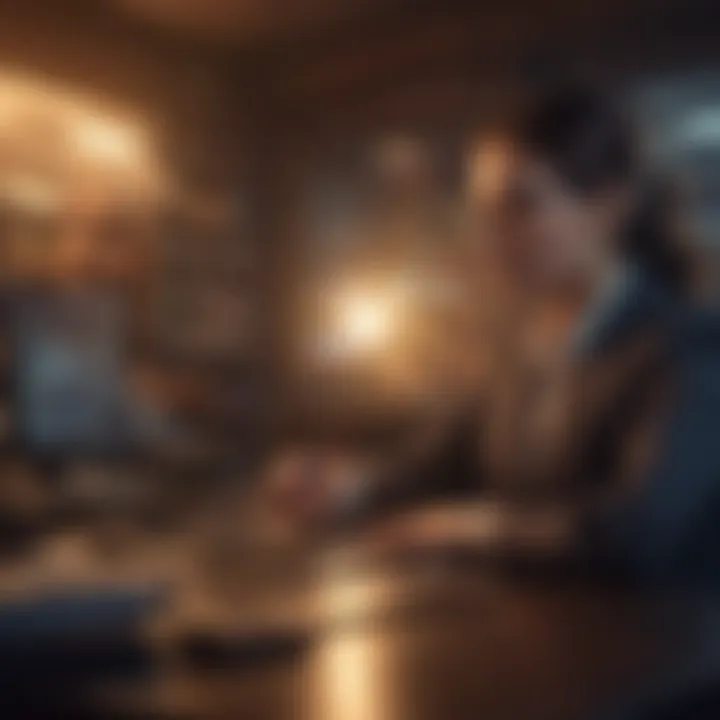
Additionally, data helps organizations in identifying emerging market trends. As consumer behaviors evolve, businesses that rely on real-time data analytics are better positioned to pivot accordingly. This adaptability often translates to substantial growth, as companies can innovate faster than the competition. When businesses monitor competitors and market conditions closely, they can tweak their strategies and seize opportunities as they arise.
To sum it up, the modern business landscape cannot thrive without a robust data strategy. The insights derived from data analysis contribute to a clearer understanding of market dynamics, consumer preferences, and operational strengths and weaknesses. As firms increasingly acknowledge data's role as a cornerstone for decision-making, the value placed on data analysts will undoubtedly soar, positioning them as essential players in fostering growth and navigating an ever-changing business environment.
Key Skills Required for Data Analysts
In today's data-driven world, possessing the right skills is indispensable for data analysts. These skills not only empower individuals to perform their roles effectively but also significantly influence decision-making processes across organizations. The ability to navigate complex data sets, extract meaningful insights, and present findings comprehensively are paramount. As decisions become increasingly dependent on data, the skill set of data analysts must evolve concurrently to meet these demands.
Technical Skills and Tools
Proficiency in Statistical Software
Statistical software is the backbone of data analysis. Mastery in tools like R or SPSS allows analysts to carry out sophisticated statistical operations. These software packages provide a robust platform for exploring data characteristics, understanding variability, and measuring relationships between variables. A compelling aspect of proficiency in statistical software is its ability to handle large volumes of data with precision.
The unique advantage of software like R is its extensive packages and community support, making it a popular choice among data analysts. Moreover, while these tools can seem intimidating to beginners, their strong statistical capabilities make them an indispensable asset. However, there’s a flip side; some statistical software may have a steep learning curve, which could present a barrier for newcomers.
Knowledge of Programming Languages
Understanding programming languages such as Python or SQL is crucial for data analysts. These languages allow for effective manipulation and analysis of data, which is the core responsibility of an analyst. Python, in particular, boasts a wide array of libraries, like Pandas and NumPy, tailored for data analysis. Its versatility and user-friendly syntax make it highly sought after in this field. The key characteristic here is that programming languages enable automation of repetitive tasks, streamlining workflows significantly.
One unique feature of programming knowledge is that it allows analysts to build custom solutions tailored to specific data challenges. While the learning process can be daunting, the payoff is often substantial, giving analysts an edge in making data-driven decisions. Yet, it's important to remember that relying solely on programming can sometimes lead to overlooking the importance of statistical reasoning.
Data Visualization Tools
Data visualization tools like Tableau and Power BI play a pivotal role in translating complex data into visually engaging representations. The ability to create dashboards and graphs that communicate insights effectively is a vital skill for any data analyst. The primary characteristic of these tools is their capacity to facilitate data storytelling, enabling stakeholders to glean insights at a glance.
These tools are particularly beneficial because they encourage collaboration across teams, allowing varied audiences to engage with data meaningfully. Their unique drag-and-drop interfaces make them accessible, even for those without a data background. However, a disadvantage to note is that too much reliance on visualization can lead to oversimplification, where important nuances might get lost in translation.
Analytical Thinking and Problem Solving
Analytical thinking and problem-solving are essential skill sets for data analysts. The realm of data analysis often involves tackling challenging questions and deriving solutions that directly impact business strategies. Analysts must approach problems with a structured mindset, breaking down complex issues into manageable parts. This ability to dissect and analyze complexities is not just advantageous—it is often the difference between a good analysis and a great one.
Additionally, the adaptability and creative thinking of data analysts allow them to explore multiple pathways. They don’t just answer the questions posed; they also identify potential questions that stakeholders might not realize they need to ask. This foresight can unveil opportunities that are not immediately evident. The combination of analytical thinking and problem-solving does not merely enhance workflow efficiency; it fosters a culture of proactive decision-making within organizations.
"The art of being wise is the art of knowing what to overlook." — William James
The Methodology of Data Analysis
In the realm of data-driven decision-making, understanding various methodologies of data analysis is indispensable. These methodologies provide a structured approach to interpreting data, allowing organizations to deduce actionable insights from raw figures. The importance of this section lies not merely in the variety of methods available, but also in the distinctive benefits that each brings to the table. With data in abundance, a clear methodology acts like a compass, guiding analysts toward informed and strategic choices.
Descriptive Analysis
Descriptive analysis serves as the foundational stone of data analysis. It provides a summary of historical data, giving organizations a glimpse into their past performance. This form of analysis answers the fundamental questions: what happened? By utilizing statistical tools, data analysts can paint a vivid picture of trends, patterns, and anomalies.
For instance, consider a retail business that seeks to understand its sales performance over the last year. By employing descriptive statistics, such as averages, totals, or percentages, the analyst can identify peak shopping periods or best-selling products. Recognizing these insights enables businesses to strategize better for upcoming seasons and tailor marketing efforts accordingly.
Descriptive analysis is like looking in the rearview mirror; it shows where you've been but doesn't predict where you might go.
Some techniques used in descriptive analysis include:
- Data Aggregation: Summarizing data from various sources into a cohesive view.
- Data Visualization: Utilizing graphs and charts to make data more accessible and understandable.
- Statistical Measures: Averages, medians, and modes provide clarity on data sets.
Predictive Analysis
Moving beyond just the past, predictive analysis focuses on forecasting future outcomes based on historical data. It employs statistical models and machine learning techniques that assess patterns and relationships to make informed predictions. This form of analysis operates on the principle of probability; it gives an idea of what could happen if certain trends continue.
For example, in the stock market, predictive analysis can assist investors by analyzing historical stock performance, economic indicators, and market sentiment to forecast potential price movements. If an analyst identifies a consistent upward trend in a stock’s performance during a specific timeframe, they may predict a continuation of that trend.
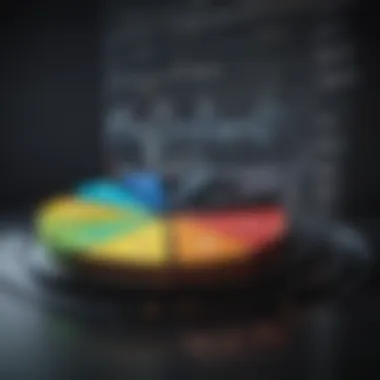
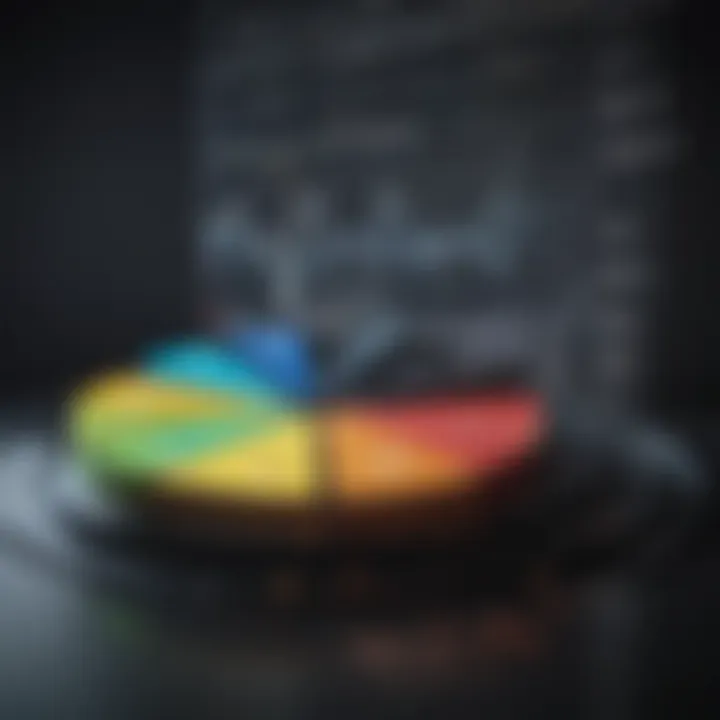
Predictive analysis employs several methods, including:
- Regression Analysis: Establishing relationships between dependent and independent variables.
- Machine Learning Algorithms: Such as decision trees or neural networks to improve predictive capabilities.
- Time Series Analysis: Examining data points collected or recorded at specific time intervals.
While predictive analysis can offer valuable insights, it’s crucial to remember that predictions are not guarantees. They serve as educated projections based on the available data.
Prescriptive Analysis
Finally, prescriptive analysis takes the findings of descriptive and predictive analytics a step further by recommending actions. It not only identifies what could happen but also advises on courses of action to achieve desired outcomes. This type of analysis can empower businesses to optimize processes, minimize risks, and capitalize on opportunities.
For instance, in operational management, prescriptive analysis can suggest the most efficient allocation of resources based on predictive data regarding future demand. In the energy sector, it can provide recommendations on managing grid operations based on predictions of power consumption.
Key components of prescriptive analysis include:
- Simulation Models: Creating virtual scenarios to test various strategies.
- Optimization Algorithms: Finding the best solution from a set of feasible options.
- What-if Analysis: Exploring the potential outcomes of different decisions in a controlled scenario.
Applied correctly, prescriptive analysis can drastically enhance decision-making, leading businesses through uncertain landscapes with a map in hand.
In summary, the methodologies of data analysis not only help organizations to make sense of their data but also empower them to act strategically, paving the way for informed decision-making in an increasingly complex world.
Applications of Data Analysis Across Industries
In today’s fast-paced world, data analysis is not just a support function; it’s become a cornerstone across numerous sectors. The way that businesses interpret, manipulate, and use data drives their growth and operational efficiency, making the involvement of data analysts pivotal. This section sheds light on how various industries harness the power of data analysis, the specific applications within those areas, and the tangible benefits derived from this practice.
Healthcare Sector
The healthcare sector has seen a vast transformation through data analysis in recent years. Hospitals and clinics are no longer just places for treatment; they are now data-rich environments where patient insights can dictate care protocols. For instance, by analyzing patient records and treatment outcomes, healthcare providers can determine which treatments yield the best results for specific conditions. This can save lives and optimize resources.
Consider predictive analytics in patient care. By leveraging historical patient data, healthcare institutions might predict sudden patient deterioration, allowing timely intervention. Moreover, public health initiatives thrive on data – from monitoring disease spread to evaluating the effectiveness of vaccination campaigns, every piece of analysed data plays a part in informed decision-making.
Financial Services
In finance, data analysis is king. banks and investment firms utilize it to assess risks, detect fraudulent activities, and predict market movements. Analysts dive deep into stock market data, economic indicators, and consumer behavior analytics to guide investment strategies. With real-time data processing, institutions stay a step ahead, ensuring their decisions are not only informed but also rapid.
For example, credit scoring systems rely heavily on data analysis to evaluate the creditworthiness of individuals. Data analysts can sift through varying economic factors and the financial history of an applicant, leading to fairer and more accurate lending decisions. This kind of meticulous analysis helps maintain the stability of the financial ecosystem and offers critical insights into potential market disruptions.
Retail and E-commerce
The retail landscape has been reshaped through data analysis as well, particularly with the rise of e-commerce. Retailers leverage data to comprehend customer behaviors, preferences, and purchasing patterns. Analyzing these trends enables them to tailor marketing strategies towards specific audiences, increasing conversion rates dramatically.
For instance, companies like Amazon analyze vast amounts of browsing and purchasing data to provide personalized recommendations for their users. This not only enhances the shopping experience but also significantly boosts sales. Likewise, data analysis helps retailers manage inventory effectively, preventing shortages or excess stock.
"Data is the new oil"– this rings particularly true in retail, as the ability to analyze data translates to competitive advantage.
Overall, from healthcare to finance and retail, the significance of data analysis spans a broad range of applications. Industries are realizing that harnessing this strategic asset is not just beneficial but essential for staying ahead in a data-driven landscape.
Ethical Implications in Data Analysis
In an age where data reigns supreme, the role of ethical considerations has become paramount in data analysis. Data analysts wield the power to draw insights from seemingly chaotic numbers, but with this power comes a hefty responsibility. It’s not just about crunching numbers; it’s about navigating a landscape fraught with potential pitfalls, including privacy concerns and bias in interpretation. As we continue to depend on data-driven decisions, understanding these ethical implications is crucial to ensuring that the trajectory of progress leads to equitable outcomes.
Data Privacy Concerns
Privacy is a hot-button issue in today’s digital age. Every click, purchase, and interaction online generates data. Yet, beneath the surface lies a swirling tide of personal information, much of which needs careful handling. Data analysts are at the crossroads of this intersection; they must tap into valuable insights without trampling on individual rights.
Data privacy concerns stem from the unauthorized access or misuse of personal information. For instance, in analyzing customer data, an analyst might uncover buying habits and preferences. If this data is shared without consent or exploited for shady purposes, it can lead to breaches of trust. Just imagine receiving targeted ads that seem to know too much about your personal life – unsettling, right?
To mitigate these risks, data analysts should put privacy by design into practice. This means implementing safeguards throughout the data lifecycle, such as:
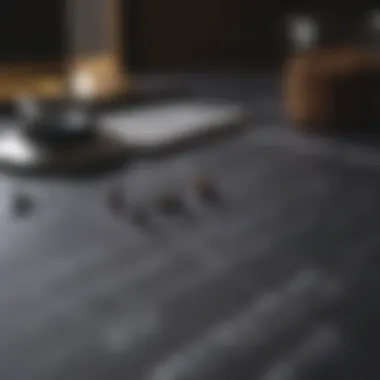
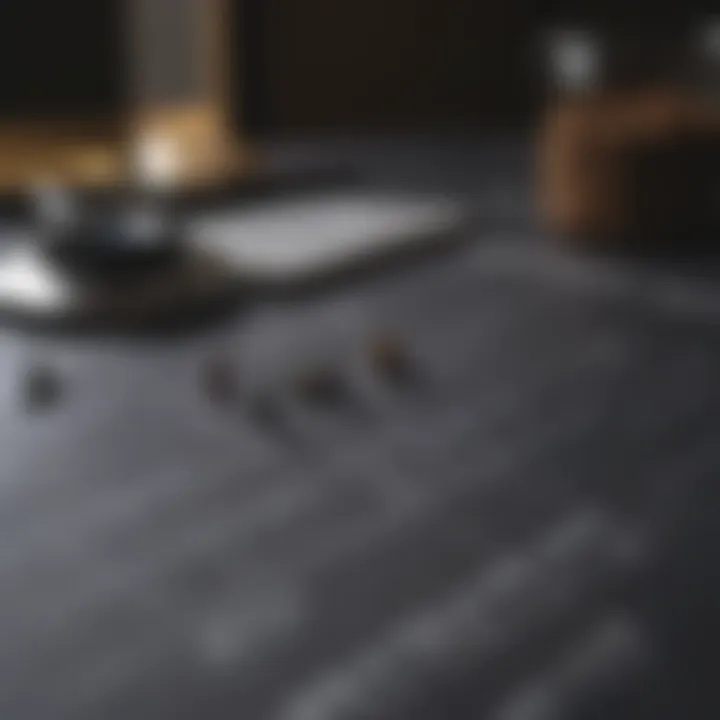
- Ensuring data anonymization so individual identities are protected.
- Obtaining explicit consent before using personal information for analysis.
- Staying compliant with regulations like the GDPR, which enforce strict rules on data use.
The importance of data privacy cannot be overstated; a breach can tarnish reputations and lead to significant financial repercussions.
Bias in Data Interpretation
Another major pitfall is bias, which can sneak into analysis in ways that can twist outcomes into something unrecognizable. Bias arises when data is selectively interpreted or when there’s an unintentional influence on the findings based on personal beliefs or societal norms. This can manifest in various forms—be it through sampling bias or confirmation bias.
For instance, if a data analyst predominantly analyzes data from a single demographic, the conclusions drawn could inadvertently reflect that specific group’s perspectives while omitting the broader population’s voice. In the realm of financial analysis, this could lead to strategies that benefit a select few instead of promoting inclusivity across markets.
To combat this bias, consider the following approaches:
- Diverse Data Sources: Use varied datasets to include different demographics and viewpoints.
- Double-Checking: Encourage peer reviews of findings to spot potential biases before conclusions are drawn.
- Awareness Programs: Continuous education on bias can help analysts recognize and mitigate its effects.
"In the world of data, every stone left unturned could lead to a skewed understanding of reality."
The implications of bias are profound—it can distort reality, impair decision-making, and perpetuate inequalities. As the landscape of data analysis continues to evolve, ethical awareness will be an essential tool for analysts, shaping the future of how we interpret data and ensuring that decisions are made with both accuracy and integrity.
The Future of Data Analysis
The role of data analysis is constantly evolving, driven by emerging technologies and market needs. As organizations increasingly rely on data to inform their strategic decisions, understanding the future of data analysis becomes essential. With the rise of automation and artificial intelligence, data analysts will need to adapt and enhance their skill sets to stay relevant.
Emerging Technologies
Artificial Intelligence in Data Analysis
Artificial Intelligence has become a game changer in data analysis. Its ability to analyze vast amounts of information quickly and accurately sets it apart. By automating labor-intensive processes, AI allows data analysts to spend more time interpreting results and advising stakeholders. Also, AI tools can uncover patterns that might elude even the most seasoned analysts, making them indispensable in identifying trends and anomalies.
A key characteristic of AI in this field is its machine learning capabilities. This feature enables models to learn from data continuously, improving their predictions over time. It's popular because the benefits are clear: efficiency, scalability, and depth of insights. However, there are considerations to keep in mind. The reliance on AI may inadvertently contribute to job displacement, where traditional analytical roles become obsolete.
Nevertheless, the advantages are notable; organizations can make faster and more informed decisions, resulting in significant competitive advantages. Essentially, AI in data analysis helps organizations keep their ears to the ground, responding quickly to market changes. But, it must be balanced with potential drawbacks, such as over-reliance on technology, which can lead to misunderstandings of data contexts.
Big Data Analytics
Big Data Analytics stands out for its capacity to process enormous amounts of structured and unstructured data. It allows organizations to glean insights from vast data sets, creating narratives that can inform critical business decisions. This capacity to harness massive volumes of data allows analysts to correlate and cross-reference information across various platforms and user interactions.
One significant aspect of Big Data Analytics is its real-time analysis capability. This enables decision-makers to act quickly based on currently relevant insights. As a result, insights derived from big data can directly influence operational strategies, customer targeting, and product development.
Nonetheless, organizations must confront unique challenges. Managing such data often requires substantial investments in infrastructure and technology. Moreover, the sheer quantity of information can sometimes lead to information overload, where valuable insights get lost amid noise. Thus, organizations must strategize on how to sift through data efficiently to find actionable insights.
Trends Shaping the Industry
As technology and markets evolve, several key trends influence the future of data analysis:
- Increased Focus on Data Privacy: With stricter regulations on data protection, data analysts must prioritize ethical handling of information.
- Integration of Advanced Analytics Tools: Analysts are likely to employ innovative tools that leverage AI and automation, streamlining processes.
- Collaboration Across Departments: Data-driven decision making will necessitate cooperation among various departments, making analysts central players in team-oriented environments.
These trends ensure that the importance of data analysts will only grow, solidifying their role in steering organizations toward data-driven success.
End
In a world increasingly driven by data, the conclusion of this article highlights the pivotal role that data analysts occupy in shaping modern decision-making. As organizations navigate the complexities of the digital age, their ability to transform vast amounts of data into actionable insights becomes not just a luxury, but a necessity. Data analysts serve as the interpreters of this information overload, translating numbers and figures into strategies that propel businesses forward.
Recap of the Importance of Data Analysts
- Strategic Decision-Making: Data analysts provide critical insights that inform strategic choices, empowering businesses to align their goals with real-time data trends.
- Enhanced Operational Efficiency: Their work enhances internal processes, identifying inefficiencies and suggesting improvements that can save both time and money.
- Market Understanding: Through analysis, these professionals shed light on consumer behavior, helping companies understand market dynamics and adjust their offerings accordingly.
Effectively, data analysts act as navigators through the chaotic sea of data, easing the journey toward informed decision-making. Without their expertise, organizations risk sailing blindly, vulnerable to the currents of unpredictability that characterize today's marketplace.
Final Thoughts on Data-Driven Decision Making
Embracing a data-driven approach to decision-making is no longer an optional strategy; it’s a prerequisite for survival and success in a competitive business landscape. As established in this article, the contributions of data analysts cannot be overstated. They provide clarity amidst chaos, a lighthouse that guides firms toward strategic objectives. Their work not only enhances individual company performance but also drives broader economic growth by fostering innovation.
"In the end, data is not just an asset, but a foundation for building a sustainable future in any industry."
As financial enthusiasts and individual investors evaluate and engage with companies, recognizing the value that skilled data analysts bring to the table will influence their own decisions, ultimately underscoring the symbiotic relationship between data analysis and successful, informed decision-making.